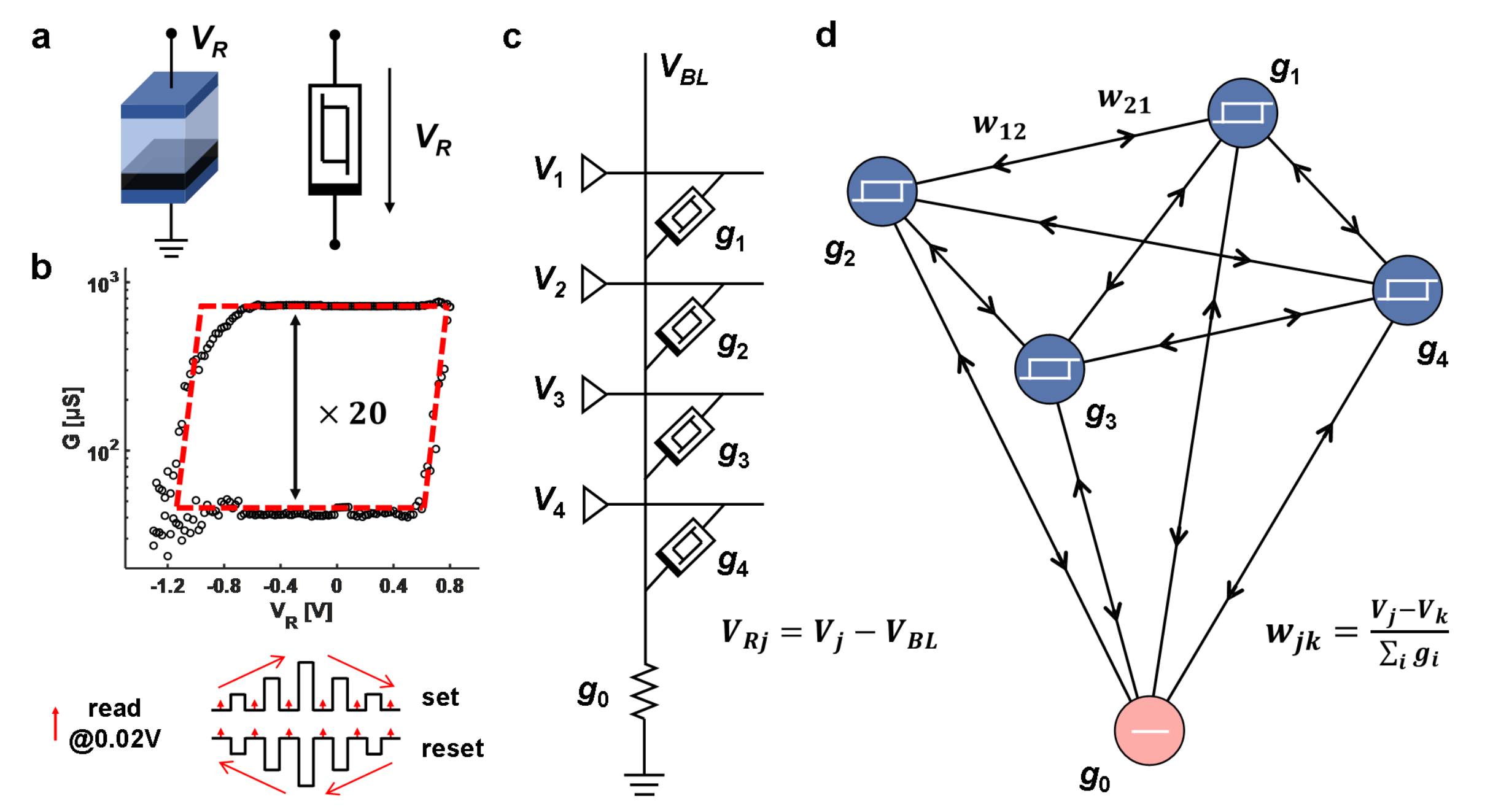
Fig.1. Model of a memristor circuit
Peking University, October 14, 2024: Fundamental to the development of artificial intelligence (AI), associative memory models of neural networks are designed to store and quickly retrieve information based on connections between elements. Recently, a research team led by Peking University professor Sun Zhong studied a resistive memory, or memristor, attractor network model that could overcome the limitations of the original Hopfield network model, which was recognized by the 2024 Nobel Prize in Physics.
The team proved for the first time that a circuit composed of a column of memristor devices is essentially an attractor network, which can be used in associative memory and combinatorial optimization. Their findings were published in Nature Communication on September 12, 2024.
Why it matters:
In the classic Hopfield network model, the storage capacity increases linearly with the number of neurons, so the network can only remember a small number of states at the same time. Increasing the storage capacity can be realized by using different nonlinear activation functions or providing different weight configurations, but hardware implementation has not yet been explored.
Memristors are bidirectional and nonvolatile neurons that can store more stable states in the circuit, greatly improving the storage capacity of associative memory. Thus, a resistive memory network allows for more stable states stored. This could be a highly compact and efficient solution for associative memory and is conducive to the development of new attractor network models or hardware solutions.
Fig. 2. Comparison between the resistive memory network and Hopfield network
Methodology:
The research team fabricated resistive random-access memory (RRAM) devices and conducted experimental demonstrations of the recurrent neural network (RNN) model of a memristor circuit. An energy function for the probabilistic model of the memristor network was also constructed, and the team performed proof-of-concept demonstration for associative memory applications.
Key findings:
-Based on the thresholded, recyclable and nonvolatile switching characteristics of memristor devices, the team demonstrated that a circuit composed of a column of resistive switching devices is inherently an attractor network. A nonvolatile memristor device is an artificial neuron that provides a hysteretic nonlinear activation function.
-A memristor-based attractor network facilitates associative memory applications with less hardware cost, as compared to the conventional implementation of the Hopfield network where resistive devices are used as synapses and voltages represent neuronal input/output.
Fig. 3. 4-neuron circuit for associative memory application
Source: Nature Communication
Edited by: Wu Jiayun